1. Satellite Data

Satellite images from the European Space Agency (ESA) are publicly available and have a medium resolution (10 m per pixel) that allows us to detect where deforestation is happening even with the naked eye.
Optical images are easy to understand and to process. However, it suffers from cloud occlusions that prevents the light to reach the ground. To handle this, we can also incorporate Synthetic Aperture Radar (SAR) images, which are not affected by clouds. They are taken by an active sensor that sends microwaves to the earth and measures how long it takes for each wave to come back. This way we can create a map with high geometrical details of the ground. We aim at combining the best of both, Optical and SAR images to detect where deforestation is and will be happening.
2. Direct drivers of deforestation
Industries like agriculture, logging, and urbanization are the mayor drivers of deforestation (Kissinger et al., 2012). We can fortunately see these direct drivers using satellite data. It is however a large amount of data that can’t be processed manually. Therefore, we can exploit the recent success of AI, that allows us to process this huge number of images to extract precise information about forest changes and raise alerts of when and where trees are at risk of being cut down.
3. Artificial Intelligence
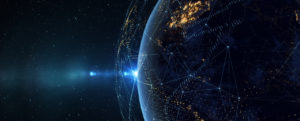
AI is fundamental to develop new automated tools to understand the world around us. This is why we want to put it at the service of tropical rain forests and raise automated alerts of where deforestation could be happening. Using Neural Networks and historical imagery of how deforestation has happened in Borneo, we can learn this in an automated way directly from the data.
Neural Networks and more specifically Convolutional Neural Networks (CNNs) are useful to process spatial data like images. CNNs became popular for example to classify the images of handwritten letters or Dogs and Cats. CNNs are learning visual characteristics (also called features) like the fur, eyes and specific anatomic differences of each animal. All of this is done in an automated way, provided we have large amounts of training examples to teach the network how a cat is different from a dog.
Building on more complex versions of CNNs, we can also teach the model how deforestation is happening, whether it is due to logging in the area, human expansion or palm oil fields nearby.
We can use these models to process the 743,330 km² of Borneo in a heartbeat and raise alerts of where forest should be saved.
4. Going beyond direct drivers

A more difficult task is to understand where deforestation could happen even before we see roads of logging or cities growing. For this reason, we need to analyze how the economic and social context of the island like commodity prices of Palm Oil (highly produced in the island) and population growth will increase the probability of deforestation in the area. Fortunately, we can push our AI models to incorporate further data sources (like market prices etc.) to extract information that goes beyond what is clearly visible in the satellite images. We expect to find patterns that will allow us to predict where future deforestation is likely to happen even before we see the traditional indicators in the satellite images.